This used to be called Data, Kittens & Economics but I realized - I don’t know much anymore about economics. I have some ideas, but they are mostly political, and far from scientific. It’s time for some change. Welcome to Data, Kittens & Society.
“ci avete fatto caso che i lavoratori dello spettacolo sono lavoratori?”
~article from an Italian political magazine, “Ossigeno”.
“Did you realize that workers in the field of entertainment are workers?” on the economical mistreatment of entertainment workers like writers, performers, poets, cultural figures.
My comment over the big Apple regulatory problems in the EU is that … I mean, they have no issue in bending every which way the Chinese government wants. Do they?
(and the bends they want are quite sadistic I must say.)
What Instagram has caused in internet dance videos is to have the hardest moves at the start of the choreography so if they come out wrong you can quickly stop and start the video again 😾🎭
Not sure if its me, due to lately me being even farther from centralized social media than usual. But I’m seeing a bit of a shift in perspective. It feels like more people are realizing that the online image of our society is fake and the one that matters is outside. 🤞
This very nice coffee was served to me in a rather surprising establishment.
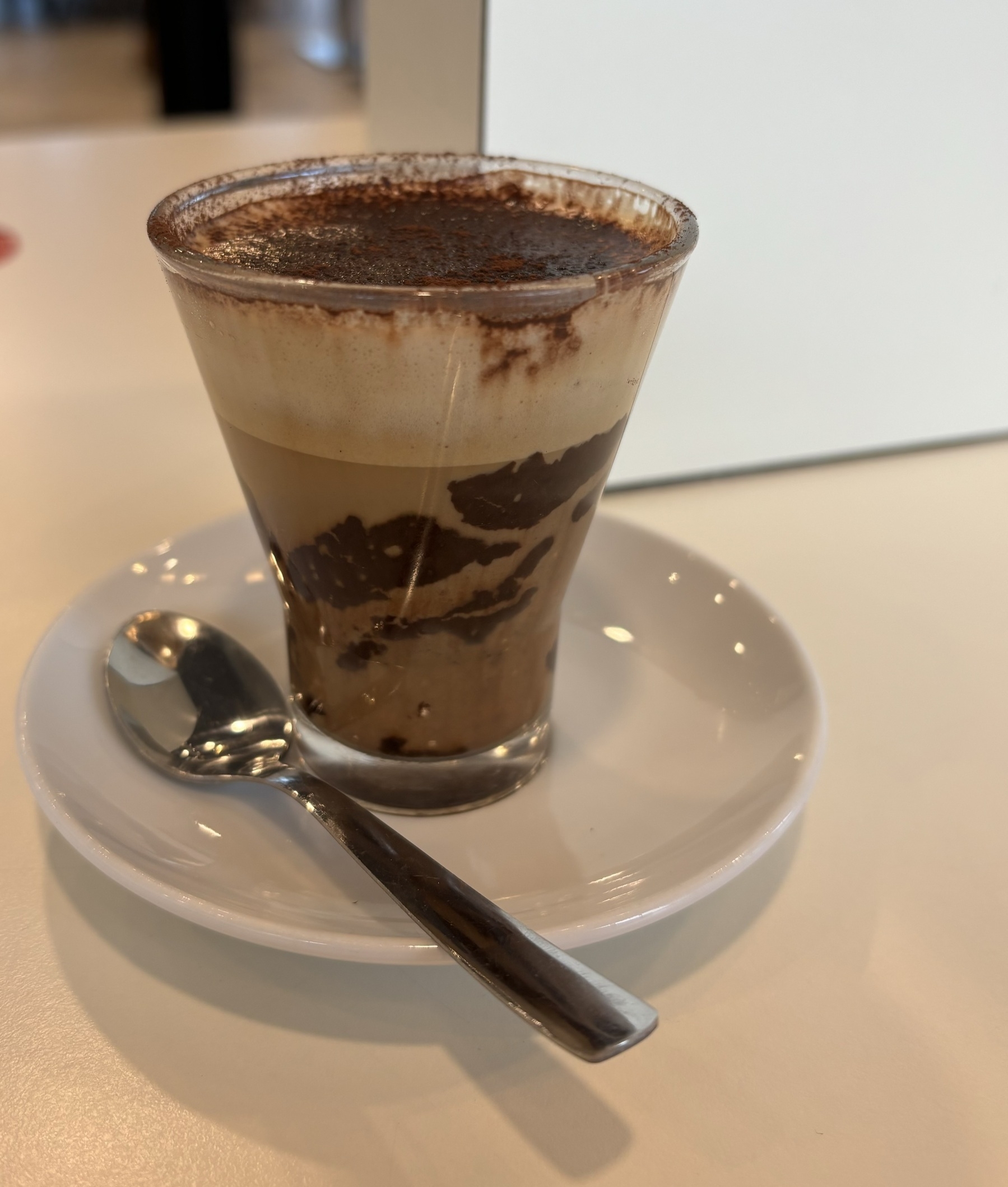
Note to myself: opening more email addresses to solve the email problem is not the answer
👋 Micro.blog - what did I miss these last few months? ☺️
I’m starting to be pissed at heteroamatonormative tv shows. 🤣 Suits is sooooooooooo full of basic boys.
Looking to start a debate. I think governments should absorb the fluctuations of energy prices during the 4 seasons. Or else what, you can only be warm at home during winter if you have the cash?
Generally not a fan of action movies but I’m an enthusiastic fan of John Wick. I found the fourth lacking in either the stunt choreo or the performers, but the rest looked like awesome cinematography to me.
Today I had the experience of understanding a bit of the mind of a right-wing populist Italian voter. They also, like me, are worried and disturbed about the house rent prices going to the moon, just like the left is. However, they attribute the reason for that to “influencers coming down from Milan and having lots of money to rent here, which makes the prices go up”.
It’s a theory. Reminds me of those in economics that focus their attention on the side of demand instead of the side of supply/production. I’d say the prices are not made by the demand. They, particularly in the house rent sector, are made by the supply side due to their overwhelming strength in a sector that is based around a fundamental need. House owners are way too free to make up prices completely without check, sometimes forcing people who live somewhere because they are working or studying there, to move further from the city, further from their place of work or study.
Yes, the problem of what I call plastic tourism for sure needs to be discussed and addressed. However, blaming the exorbitant rent prices on rich influencers from Milan (when we don’t even live anywhere close to Milan) seems really naive and to me feels like a distraction from the real problem. House owners asking for thousands of euros a month and taking advantage of workers or young students who can’t refuse because they need a house somewhere near their place of work… Makes me icky and sick.
What’s happening between palestine and israel is very real and very serious. I’m not a fan of rockets, colonization, brutality, injustice, state crime, human rights violations. Not a fan of any of that. What do you do then when you get the worst end of the stick every single time?
I don’t understand. Do juries from jury trials get paid at least a minimum of daily living expenses in those countries that have such judicial processes? And if no, how … ? How is it possible?
How exhausting must be to work as a fast food cashier/server. She’s taking my payment while taking the next client’s order in her headphones.
“Did you make a noise?
Was it you who made the noise?”
My roommate Bolle! He’s 2 and 4 months and he’s been with me for the last 2 years.
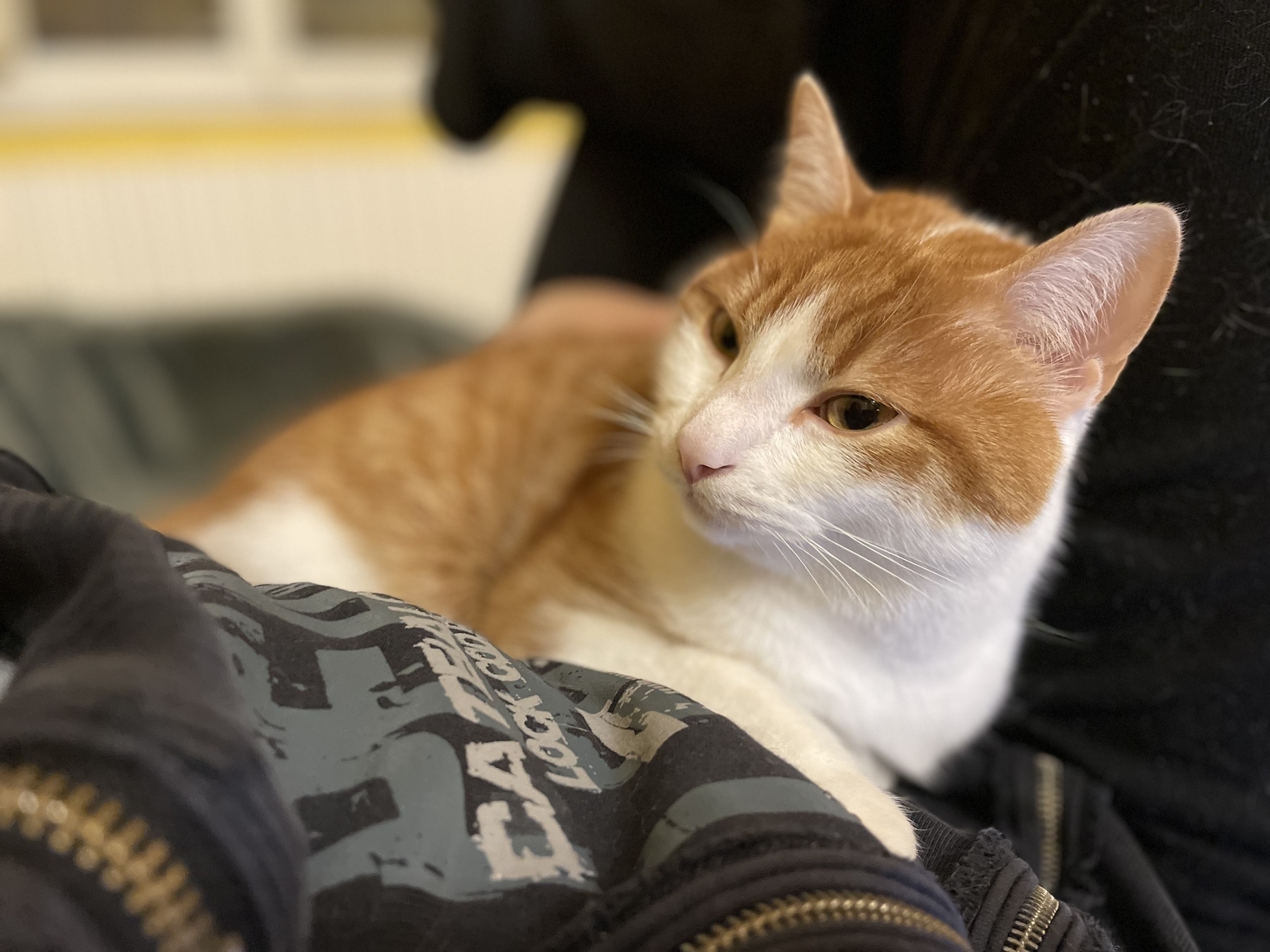
Isn’t expat just another word for immigrant? According to Oxford’s…
immigrant | ˈɪmɪɡr(ə)nt | noun
a person who comes to live permanently in a foreign country
expat | ˌɛksˈpat | informal noun
a person who lives outside their native country
So why do the two sound so different and why are they used so differently?
Notes on machine learning, part 1: What is it
This is the first part of a series (that’s the intention at least 🤣) trying to give an overview, from a broad lens, of what I do in my academic field. It’s machine learning - ML1 - and in this I’ll focus on what it is, while I’ll talk about its applications, research and development in later parts. Keep in mind also that this is the first time I write extensively about what I do in general terms and in a popular format! I’ll explain mainly through examples to try and avoid as possible any formal definitions. I’ll try my best 🙃 and please send feedback, it’ll help!!
ML can help in many fields but the problem to solve often boils down to a prediction. Some pretty famous examples of usage are:
- how will the rates of infection from Covid develop over specific locations in the following weeks and months?
- which advertisements to put in front of a specific user’s eyes to maximize the chance that they will stop scrolling, click and buy?
- how does my iPhone correctly identifies its owner’s face and unlock?
- can we estimate future rates of reoffending for people convicted of crimes?
- how to evaluate workers performance in any given field?2
There are also less popular and yet not less interesting applications, such as:
- how will a community’s population evolve in the coming years, in regards to phenomena like gentrification and segregation?3
- how to help doctors in making more informed diagnostic and treatment decisions?
- how to ensure a prediction is made for ethical reasons?
- how do we isolate, recognize and measure unfairness?
Classical predictive models
There are several ways to try to predict the future. A few common ways are:
- based on human-set rules,
- based on models of reality,
- based on statistical knowledge of historical behavior.
Let’s look at each of them because ML draws concepts from each.
Human-set rules
This is what I refer to as the “classical” way of controlling and predicting behavior in artificial systems. An example would be: if the industrial machine for baking cookies reaches 90 C° on the outside surface, shut it off automatically before it burns. This rule (90 C° external sensor → shut down) is set in this example by a human expert. They know that:
- 90 C° is above the normal temperatures during usage, and
- it’s far enough above the normal to be dangerous to the materials used or to the factory in which the machine is installed or to the humans operating it.
There are fields in which this approach is the gold standard, and probably should be so for a long time (e.g. nuclear power plants control systems). Still, this approach represents some of the roots of ML.
Models of reality
Many engineering design works are first represented in a computer model through programming and design languages. For instance, a highway bridge will be represented in a computer before being built. The same will happen during the design of a space rocket4. The softwares employed allow for artificial perturbation of conditions, like introducing strong winds or earthquakes to check and see what would happen to the bridge as designed.
Statistics and historical behavior
There are several assumptions that we make when trying to infer conclusions from historical behavior using statistics. I won’t talk about all of them here, just one: the concept of a “data generating machine”. The idea is that phenomena are directed by invisible, highly complex mathematical functions that for a set of values of variables (the input of the function) give an outcome to the phenomena (the output of the function).
A (made-up) example
Variables |
---|
number of trains passing on the same tracks today |
number of passengers for each of those trains |
detailed weather characteristics |
experience of train staff including conductors |
Generates |
---|
number of minutes a train will be late at each station |
Once again this example is 100% made up. All I’m trying to picture is the hypothesis, made in statistics, of the existence of a specific relationship between variables (the characteristics of the causes of the phenomenon) and the outcome of the phenomenon itself (in this case, how many minutes the train will be late). The idea of a data generating machine is that there exists a maths function that formalizes this relationship and assigns unique values of the outcome to each set of inputs.
Much of the predictive statistical modeling work is to try and figure out this function5 as accurately as possible. There are many possible techniques. We’re getting closer to machine learning.
Inference of an approximate function
A regression is one of these statistical predictive techniques and is used to extract this function from a set of data. The data consists of sets of variable values and outcomes. We call the variables “features” and the outcome “class” or “dependent/target variable”6.
Another example
Let’s suppose that the phenomenon in question is the causal connection between the number of years of formal education that a person had and their current monthly revenue. We’re hypothesizing that the first determines the second. These might be the data we have (made up, but realistic7):
The process of applying regression might extract this function, in pink:
This function does not provide, for each value of the feature, values of the phenomenon that exactly mirror the input data. Instead, for each value of X it provides the value lying on the segment drawn. It’s just the best function that can be inferred from the data given the algorithm chosen by the operator; in this case, the algorithm is a “linear regression with 1 regressor and the OLS optimization function”.
A deep dive on linear regression is here in a 30 minute really well-made video by a fantastic YouTube channel, StatQuest.
Machine learning predictive models
All of these three concepts play a role in ML. Applying ML to a predictive problem means all of the following:
- Modeling reality, that is creating a model of reality - although the model is automatically inferred instead of being intelligently designed by the operator;
- A model that is (often) based on rules - although:
- the rules might be so complex they don’t make sense to the human, or
- the rules might look like different types of rules than what you would expect8, or, finally,
- the rules might not look like rules at all.
- A model whose behavior and/or rules are (often) inferred from statistical, historical data9.
The purpose of creating this model is to try and predict the behavior of our phenomenon of interest based on the data (circumstances and outcomes, a.k.a. features and target variables) that same phenomenon generated in the past.
Next articles…
I’m thinking some interesting points to touch in the next parts are:
- what is the role of a human operator in ML?
- What are objective functions?
- What are some of the most crucial issues with this?
- What are interesting research directions right now?
- How does ML influence society?
Do let me know some points you’d like to read about, as well as any questions!! I’m particularly interested on how accessible this text was for non-experts. Looking forward for your feedback!
Further reading
- An MIT article, a bit more in depth on specific ML categories and on the relationship between private business and ML.
-
Part of the field that is sometimes called artificial intelligence, but I don’t like that denomination. ↩︎
-
In trying to paint a complete picture, I’ll mention all applications, not only the ethical ones. These last two examples, in particular, have come to the attention of the American press for their callousness. ↩︎
-
For those interested, an article announcing the publication of an interesting scientific paper regarding this very issue. ↩︎
-
In fact, there’s even a simulation game to build space vehicles. It’s Kerbal Space Program. I got it and tried it, but never got the hang of it, I’m really bad at building things. Same reason why I never got the hang of Minecraft. 😝 ↩︎
-
A function that, again, is hypothetical and might not exist. As an example, one might make the hypothesis that at the core of weather is a maths function. It’s a conjecture that we might never be able to verify, because there is no theoretical limit to the possible complexity of a function. We can exclude many functions from being candidates of generating a phenomenon, but it’s hard to prove that we can exclude all functions. ↩︎
-
One possible form of this function might look like $y = \beta _0 + \beta _1 x_1 + \beta _2 x_2$. This is a linear regression with two features $x_1$ and $x_2$ and a residual $\beta _0$. In regression the features can also be called independent variables or regressors. ↩︎
-
Source: OECD’s Education and earnings, Australia, 2020, 25-64 years of age. ↩︎
-
Example, based on geometry, on unusual measures of dissimilarity, or on proxy representations of reality. ↩︎
-
There are two ways to do statistical inference: one is inferring future behavior of the objects you are investigating, and is based on historical data of those same objects. The other is inferring behavior of different objects than those you have data on, but still belonging to the same population. An example is inferring the behavior of all voters when you have data (surveys) on just a smaller group of voters. In this article I spoke on the first of these two approaches, but much of the same concepts apply to the second approach. ↩︎
I see so much misinformation around AI on Linkedin. Blanket statements like “a human can solve a problem in 5 minutes; AI can solve 10 in one minute” are so disingenuous that I don’t know if it’s malice at play or just simplicity. 🤷♀️ is the algorithm just messing with me?
2 days ago, peak of 35° Celsius during the day. Today, the maximum is 24°, next week will be again 34°, but we still trading futures on oil and water 🤷♀️ kinda dumb tbh.
Ah, I just caught myself just before hitting send on a rage-reply to a Facebook comment from someone very deeply and maliciously misinformed. The power of social networks looking for user engagement at any cost, no matter the quality of that engagement, is breathtaking to me
Nice implementation of pronouns by Apple. Next step will have to be to share pronouns with third-party apps at execution time only. So the app programs in a token in Swift that is a placeholder text for the pronoun. At execution time iOS swaps in the actual pronoun. (via @pratik)
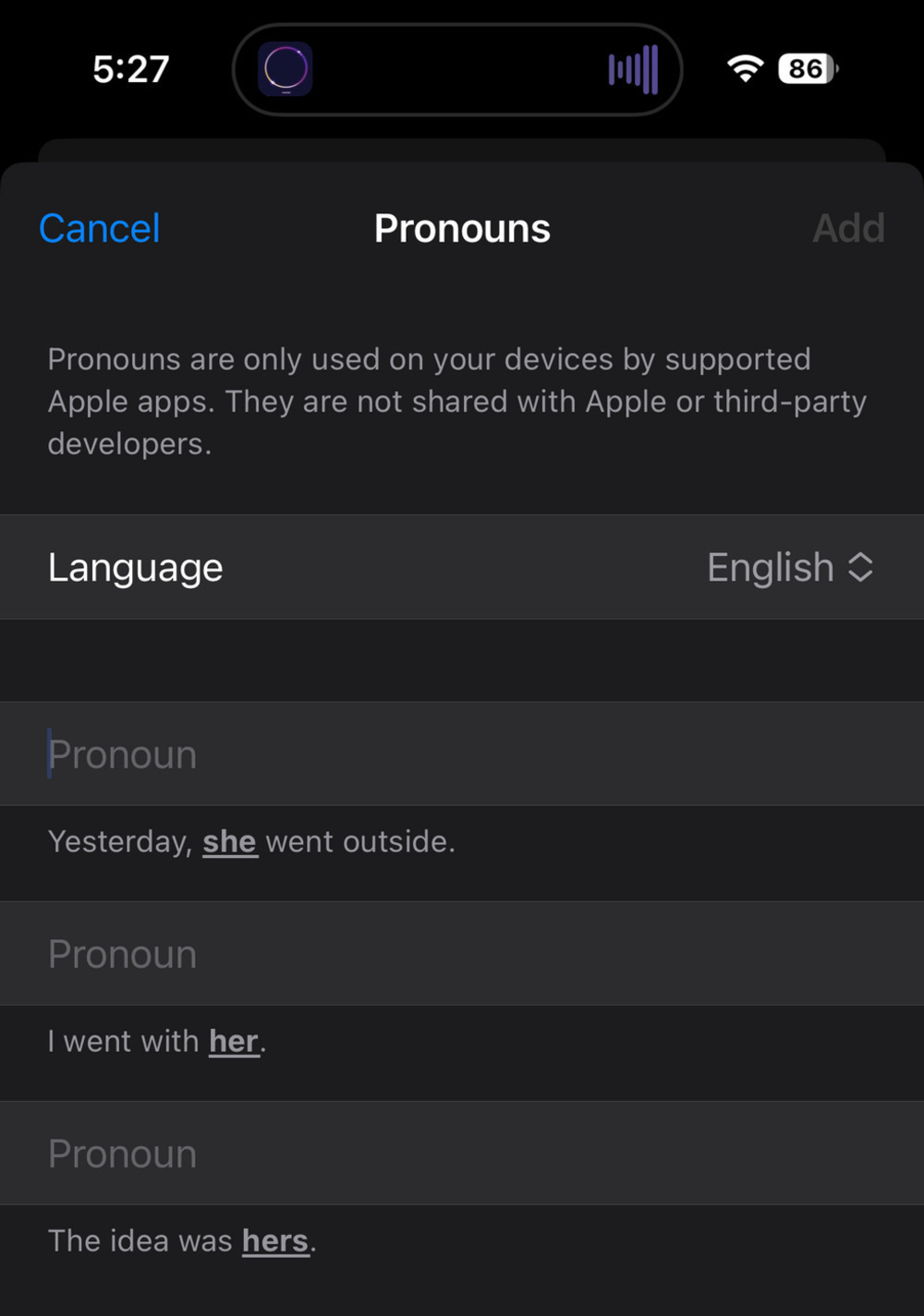
Why I don’t call what I do “artificial intelligence”
While I use machine learning, ML, or sometimes applied or computational statistics to describe1 what I do, the term artificial intelligence, AI, is now very widespread in society, industry, politics and marketing. In Italian too, where the shorthand is very similar: IA. However, I try to refrain from using it as much as possible. Why? For coherence, cultural and societal concerns.
AI is overused and already characterized in literature and cinema.
While we like to think that everyone now knows what we do, we’re nowhere near that place. There’s still millions of people who, when hearing artificial intelligence, think of machines in The Matrix. They think of consciousness, of AI-human wars, even of AI being alive. They know that AI is either malevolent and something to stop or a benevolent force that will consciously help humanity. It sounds silly? A Google engineer sometime ago thought that their chat model was alive, and recently a letter by a few AI practitioners foresaw a danger of a war against AI.
AI is already very characterized in pop culture and what we’re doing has nothing to do with that.
I don’t think what we create qualifies as intelligence.
The question of “what is intelligence” is philosophical in nature and possibly will never have one uniquely correct answer. For me, the main component of intelligence is creativity. When we see something that’s scolding hot, and we want to move it, we might poke it with a stick, protect our hand with a thick glove, kick it quickly so that our skin is not burned, or more. We might even come up with something that nobody ever did, ever. We all find it strange to think that something so simple might have a historically unique answer, but in the end, everything that exists was first made by someone, or a team, for the first time ever.
A computer isn’t able to create. If nobody ever did something, a computer won’t invent it.2
And I’ll hazard a prediction: a computer will never be able to actually be creative. Of course, what it means to be creative is also a philosophical question. Painting something beautiful used to be used as an example of creativity, but a computer can emulate it by using known painting and image patterns, rearranging them randomly or according to a distribution, joining different known techniques and patterns in a new way, and it ends up that not every painting is actually an act of creativity. Who would have guessed?
Of course there are more components to intelligence. Memory, the ability to learn from knowledge and experience, the ability to make calculations. And while the computer obviously has memory and calculation power, the ability of a computer to learn I would reckon is nowhere near the way that a human learns. A computer learns, when we’re getting to the nitty gritty, to optimize with math and statistics a mathematical function. What is that? Who chooses the function? Who chooses the metrics? Who chooses the datasets and the algorithms? Humans do, because that requires real reasoning, real intelligence.
And while there are some parallelisms between machine learning and the sociology of human growth and human acquired behavior - where it is encouraged or discouraged by the social groups we find ourselves in - I still think there’s way enough difference to see the two processes of learning as deeply different.
Much of the human learning process has to do with rewards, the human necessity of belonging, of social recognition, with the very human emotion of fear, of love, with the existence of death.
Can rewards and punishments be emulated well by mathematical functions? I don’t know. Maybe? Possibly not, possibly never? But not today, for sure.
It makes it sound like AI is not the work of humans, or that the results of AI are not the work of humans.
This is crucial. An AI denied your mortgage application? No, a human did that. Most likely a team. We as machine learning developers and data scientists need to own the results of our work. Especially its deficiencies, especially its biases, its idiosyncrasies, its reinforcements of historical unfairness. Just as well as its successes.
When we train our models on historical data without accounting that historical data paints a picture of an unfair world, then our ML models will replicate that unfairness. Experts know this all too well, in fact it’s taught in data science courses. Computers don’t have ethics, they don’t see the bias themselves. They don’t know what discrimination is, and even if we taught them that (again, with mathematical functions3), it is only humans that can tell a computer that discrimination is bad. Is adherence to the optimization of mathematical functions the same, or will it ever be the same than a fair mind, empathy, the experience of pain and the hope for a better future? Maybe. Maybe not, maybe never. But for sure it is only humans that can tell an algorithm what to optimize.
Who creates the content?
The only reason ChatGPT is able to write your college essay is that it has read billions of college essays. So if the only way that AI can produce results is based on humans’ work, is AI really anything at all without the human experience? I would argue, not.
In fact, it is the developers and financiers of ChatGPT that are writing your college essay. And all the millions of people that authored those billions of pieces of original work.
My conclusions
What I think should really be at the forefront of social discussion is the impact and consequences of AI. The European Union - following the GDPR work in privacy4 - is doing massive work in AI regulation which looks to be a good step forward, but this discussion cannot be left to experts only. We need to decide as a society how and in what direction to employ our collective efforts. And the place of experts is to educate, yes, but most importantly to own our work, its results and its impact.
We are data scientists developing machine learning algorithms. We are the artificial intelligence. And - we are not so artificial ourselves, and our computers are not so very intelligent, at all.
-
Not much of a description, yes. As I am proof reading this, I’ve realized my next post should probably be “How would I describe what I do?”. ↩︎
-
An exception is, if we asked a computer to list 1 million things that could work for moving something scolding, and then looked at those million ideas, there may be something new, but not because of intelligence, but because there were 1 million minus one silly ideas. ↩︎
-
Today, it’s not even clear how we would model discrimination and make it part of our loss functions. I read some really cool ideas though. ↩︎
-
Work which, while good, is not perfect at all. Already it looks like the anti-unsolicited marketing communications is being hollowed out by a legitimate interest interpretation that almost completely empties all the GDPR’s protections against personal data usage for commercial reasons. ↩︎
The introductory video to Apple’s WWDC has a developer throw himself off a roof while happily chasing a bubble. Hi Apple. Hello. 🤣
Coming out as social scientists and admitting that AI poses risks shouldn’t be taboo. Risks are real. Personally, more than scenarios a-la-machines in the Matrix, I’m most worried about AI perpetrating historical unfairness and AI applying decision methods incomprehensible to people. We’re working to avoid that. And I would in fact like a wide agreement by AI practicioners to engage in trustworthy applications and research.